Final presentations third AI for Health course
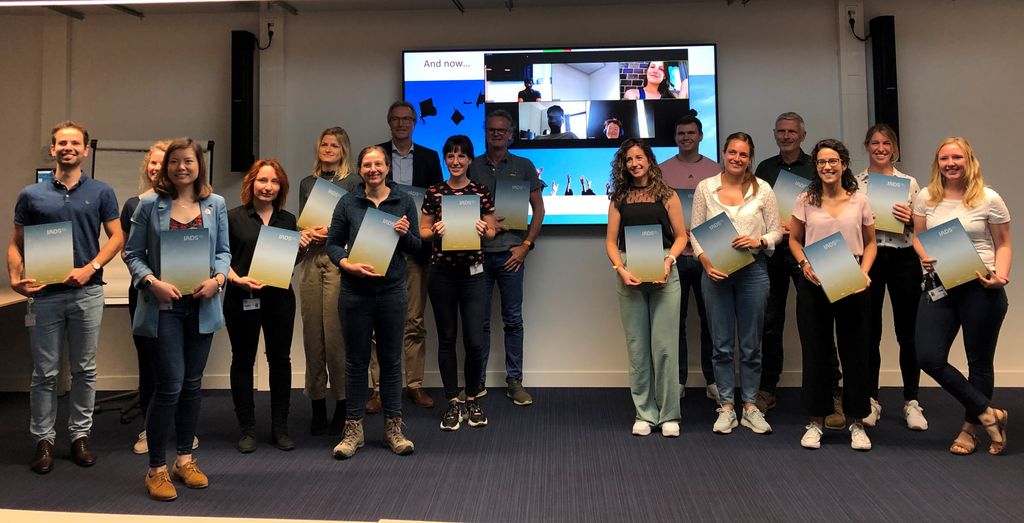
Friday, July 1st, 2022, the third AI for Health course concluded with a meeting where all participants presented their AI projects' results. About 25 Radboudumc employees and external health professionals from different backgrounds followed the third course. The fourth edition is scheduled to commence in February 2023, if you are interested, please send an e-mail to aiforhealth@radboudumc.nl.
Radboudumc organizes the 16-day course together with the Jheronimus Academy of Data Science (JADS) in Den Bosch. As part of the course, teams of participants perform an AI project. The presentations of these projects demonstrated the diversity of AI applications in healthcare.
Erik Hazenberg, Floor Wilting, Niklas Bruse and Dominique van Midden worked on the Detection of bacteria growth using deep learning. Inspecting petri dishes for bacterial growth is very time consuming due to the high amount of dishes that are produced daily. Making this daily inspection of up to 5000 petri dishes more efficient could lead to great savings in time and resources. Using deep learning, the team created a very reliable algorithm that could detect growth versus no growth on the petri dishes, thereby helping lab analysts to focus on the petri dishes that have shown growth. Further development of the algorithm should lead to better quantification of the amount of growth and the type of bacteria detected.
Sanne Vermorgen, Megan Schuurmans, Evelien van Genugten, Pui-Yuen Li and Leslie Tessier worked on Lymph node screening using deep learning. Lymphoma's are rare types of abnormalities that can be found on regular inspections of lymph nodes by a pathologist. Because of it's rarity it is hard to detect for pathologist that are not specialized in hematologic pathology. The team developed an AI algorithm to distinguish between lymphoma and reactive lymph nodes. While showing promising results, further development is necessary to make it suitable for clinical use.
Machteld van Beusichem, Ashok Selvaraj, Haras Mhmud and Thijs Kerstens worked on Detection orthodontic landmarks on 3D scans. For the detection of various orthodontic interventions, orthodontists rely on 3D scans of the teeth. By manually placing landmarks on these 3D scans the severity of e.g. an overbite can be determined. Since doing this manually is time consuming, the team has developed an deep learning algorithm that can automatically place these landmarks.
Walter Van der Weegen, Marta Bravo de Moreira Pinto, Joyce Nijkamp and Susan Lassche worked on Predicting hip treatment for osteoarthritis. Patients with hip complaints have several options for treatment with surgery being the most drastic. Which treatment is best for which patient is difficult to determine. The team has worked on a prediction machine learning model to determine the best treatment for a patient based on a questionnaire.
Liesbeth Hunik, Ilse Willemse, Yingxin Yu and John Hermans have worked on Predicting diagnosis in primary care. Primary care providers have a great challenge in determining whether symptoms might be signs of a severe illness or just a common cold. The team has worked with a large database of patient contacts with primary care givers with their associated diagnostic outcomes to create a machine learning prediction model that predicts the underlying diagnosis of symptoms during first contact.
It was great to see all partipants dive into the AI topics with enthousiasm and we hope it will provide them with new opportunities in their work.
← Back to overview